Artificial Intelligence (AI) is evolving rapidly, bringing new paradigms and redefining how systems interact with their environment. One of the most intriguing discussions in this space revolves around AI agents and tools, two concepts often used interchangeably but with crucial distinctions. Understanding these differences is vital for businesses and technologists looking to leverage AI effectively.
Defining AI Agents: from Theory to Modern Applications
The concept of AI agents is not new. Back in 1995, Stuart Russell and Peter Norvig defined an agent as "anything that can perceive its environment and act upon it." Under this broad definition, even a basic thermostat qualifies as an agent—it perceives temperature and acts by turning the heating on or off. However, modern AI demands a more refined definition.
A contemporary perspective, provided by the co-founder of ManChain, refines an AI agent as "a system that uses a large language model (LLM) to decide the control flow of an application." This operational definition highlights a key characteristic: decision-making autonomy.
Andrew Ng, a prominent AI thought leader, suggests moving beyond strict definitions and instead acknowledging that systems exist on a continuum of agentic behavior. In simpler terms, an AI system can exhibit varying degrees of agency—from simple tools that require human intervention to full-fledged agents capable of making autonomous decisions.
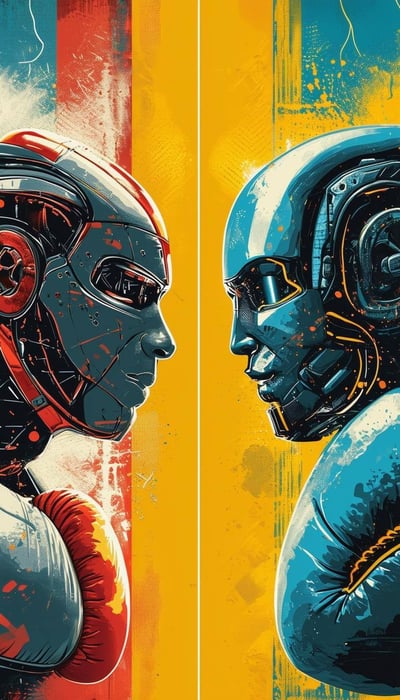
AI Agents vs. AI Tools: The Key Differences
A fundamental question arises: Where do we draw the line between an AI tool and an AI agent?
- Decision-Making: The primary distinction lies in autonomy. An AI tool requires explicit instructions from humans, whereas an AI agent can plan, reason, and execute tasks independently.
- Workflow Execution: A tool performs predefined functions, while an agent can break down complex tasks, strategize, and refine outputs over time.
- Memory & Learning: Agents can retain and use past interactions to improve decision-making. Tools, on the other hand, remain stateless—providing outputs based solely on current input.
- Interaction with the Environment: Agents can modify and respond to their environment dynamically, making them highly adaptable in complex systems.
For instance, consider an AI-enhanced database system. A tool might allow a user to query information, whereas an agent would autonomously decide which database tables to query, refine its search iteratively, and return the most relevant response.
The Power of Agent Workflows: Unlocking AI’s Full Potential
Recent research demonstrates that implementing agent workflows significantly improves AI performance. Experiments have shown that GPT-3.5 alone achieves 48% accuracy (according to the HumanEval benchmark, which measures large language models' ability to solve coding puzzles), whereas the same model integrated into an agent workflow reaches 95% accuracy.
Notably, this surpasses even GPT-4’s benchmark performance (67%).
The takeaway? Instead of waiting for more powerful models, businesses and researchers can enhance existing models through strategic workflows, such as:
- Planning before acting (breaking tasks into sub-tasks before execution)
- Reflecting on past outputs to improve future performance
- Integrating reinforcement learning to adapt dynamically
Real-World Applications of AI Agents
Despite AI agents still being in their early stages, they are already impacting multiple industries. To cover just a few notable examples:
- Healthcare: AI-driven medical assistants analyze patient data (genetics, lifestyle, and health parameters) to recommend personalized treatment plans and predict potential diseases.
- Finance: Autonomous agents can monitor markets, detect fraud, and optimize investment strategies with minimal human intervention.
- Customer Support: AI chatbots, when enhanced with agentic capabilities, can handle complex inquiries, escalate issues appropriately, and even take proactive actions.
- Education: AI tutors can design custom learning plans tailored to each student’s progress and needs.
- Robotics: The next step for AI agents involves moving from digital to physical environments—where models like those developed by Boston Dynamics could integrate advanced AI agents for real-world problem-solving.
The Road Ahead: Advancements and Limitations
Looking forward, AI agents will increasingly streamline operations, optimize decision-making, and integrate vast datasets autonomously. However, challenges remain:
- Accountability: Who is responsible if an AI agent makes a mistake? This remains a crucial legal and ethical concern, particularly in regulated industries such as healthcare and finance.
- Scalability: AI agents require real-time processing and large-scale data access, raising significant infrastructure challenges.
- Privacy & Security: Many AI applications depend on sensitive personal data. Ensuring privacy protection and compliance with regulations (such as GDPR in Europe) remains a major hurdle.
- Data Limitations: While larger datasets have driven AI progress, we may soon reach a plateau in available high-quality data, necessitating new approaches like self-learning agents trained in real-world environments.
Conclusion: AI Agents as the Next Evolution
AI agents represent a shift from static, rule-based systems to dynamic, autonomous intelligence. As businesses and researchers refine these technologies, we will see greater adoption in industries where decision-making and adaptability are crucial.
Instead of waiting for the next breakthrough model, organizations can enhance existing AI through better workflows, agent architectures, and continuous learning. The future will likely involve hybrid systems—where AI tools and agents work together, pushing the boundaries of automation and intelligence.
While challenges such as accountability, scalability, and privacy need to be addressed, AI agents hold immense potential for transforming industries and redefining how we interact with technology.